A Practical Application of Generative AI: Helping Veterans Get the Benefits They Deserve
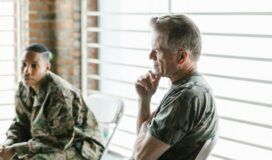
When trying to manage innumerous amounts of information, no company wants human error or manual processes to be a factor. That’s what one organization was facing, as they processed thousands of disability claims a month for the underserved and at-risk population. This labor-intensive and error-prone method needed an update. Here’s how SPR helped the company use artificial intelligence (AI) to increase the rate of claim approvals.
Why Use GenAI?
If you’ve ever played around with ChatGPT, you have some understanding of Generative AI (GenAI), a type of machine learning that uses artificial intelligence (AI) to create new content. To do so, GenAI models learn patterns and relationships from human-created content such as text, videos, images, and audio, and then use them to generate new content, which can be nearly identical to human-generated content. In business, GenAI is a powerful tool for streamlining workflows with possibilities that span all industries.
Specifically, large language models (LLM) are a type of generative AI model trained on vast datasets to understand and generate text like a human. Large language models can be leveraged for a wide variety of tasks, including virtual assistants and chatbots, content writing, and in this case: generating text responses for disability claims.
Helping the At-Risk Population Access Benefits
Our client is an organization that helps the underserved and at-risk disabled population, primarily military veterans, develop medical evidence for the disability benefits they medically, legally, and ethically qualify for from both government and private entities. To file a claim, the company collects data about a client’s specific situation and manually creates various documents to be filed together as medical evidence.
Working with thousands of clients at a given time, the journey to receiving benefits is complex, and the legacy process for filing claims is labor-intensive and error prone. Plus, it creates a “fingerprint” on the claim document that identifies the client as someone who is working with the client company. Unfortunately, this fingerprint biases the approver toward claim denial.
Our client engaged SPR to introduce AI into the process with the primary goals of reducing the labor investment and eliminating the document fingerprint, ideally increasing the rate of claim approvals.
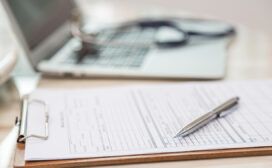
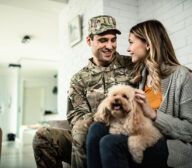
The Client’s Challenge
Currently, disability benefits defined by federal regulations are contained in a 950-page document. The process of submitting a disability claim is laden with paperwork and must meet all rules and regulations set out by governing entities. Essentially, a claim must align with specifics outlined in the government document.
As part of the company’s process, client in-take begins with nearly 2,000 questions about the client’s health history. A human employee then uses a Microsoft Word template standardized for a specific ailment or disability and manually enters information related to a client’s case. This documentation, along with supporting medical exam documentation, is then reviewed and submitted. All in all, the process is extremely inefficient and prone to error. For a simpler case, a document can take as little as 15 minutes to prepare, while for a more complex case, it can take several days. Each claim includes multiple documents.
Because of the templated system, the claim documents are distinguishable as a product of the organization, creating a so-called “fingerprint” on the document, which is seen as unfavorable for the client submitting their claim.
How SPR Used GenAI for Efficiency
We opted to use GenAI to scan the federal regulations document as well as a client’s unique health history and medical examination documents, and then generate new documentation that summarizes the client’s circumstances while emphasizing the most pertinent disability benefits required.
Leveraging a variety of technologies including AWS Postgres, S3, SQS, and EC2, and Microsoft Azure OpenAI, we created a workflow that could generate accurate claims documents in minutes as opposed to days or weeks. Real-time data pipelines were built to serve the AI workflow, which runs asynchronously so that hundreds of documents can be run in parallel. To mitigate hallucinations and ensure accuracy, AI-generated documents are routed to an employee of the organization for final approval.
The Results
Using the new generative AI workflow, the company can generate about 40,000 documents per month at about $0.50 each, resulting in significant cost savings. The documents also have more variability compared to the original templatized versions, meaning that the “fingerprint” has been removed and the claims have a better chance of being approved by the Department of Veterans Affairs.
Technology Leveraged
AWS Postgres, S3, SQS, EC2
Microsoft Azure OpenAI
Now that the company can generate these documents in a more efficient and cost-effective manner, the organization is identifying opportunities to enhance the experience and provide even more help to Veterans seeking assistance—this is what really matters.
And finally, when it comes to large language model-enabled applications, tech people often talk about this sort of use-case in theory, but few see it through to the end. Of those who talk about LLM applications, approximately 10 percent build a proof-of-concept, and of that 10 percent, another 10 percent build something that makes it to production. To work on such a sophisticated LLM application, and to successfully see it through to production, is something that our team is incredibly proud of.
If you’re ready to discover how you can harness the power of AI and LLM in your workflows, connect with one of our team members today.