Transforming Insurance Risk Management with Machine Learning
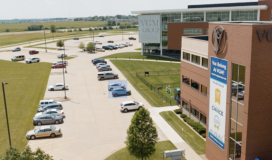
VGM Insurance, part of VGM Group, has been providing insurance solutions for professional and general liability since 1985. Over the years, the company wanted to enhance its risk management processes and improve operations. The company wanted to move from traditional, manual risk assessments to a data-driven approach.
This shift was necessary to handle vast amounts of data more effectively and make better decisions. VGM aimed to improve operational efficiency, reduce risks, and offer better services to their clients.
To achieve these goals, VGM teamed up with SPR to use machine learning (ML) to predict claim risks more accurately and efficiently.
See the Story
Hear directly from the client how we helped VGM better manage and predict the risk associated with insurance claims.
The Start of the Partnership
The initial phase involved detailed discussions and planning to align both teams on the project goals. VGM had a clear idea of what they wanted to achieve with their data and machine learning, but they needed SPR’s expertise to make it happen. The collaboration started on a strong note, with both teams ready to work together. SPR quickly understood VGM’s business needs.
“We learned that SPR had a really deep business acumen and understood the challenges,” said Scott Hagberg, Director of Technology Solutions Delivery for VGM Group. “SPR didn’t have to be insurance experts. But they understood that in business, we have processes, customers, goals and deadlines, and all these mattered. SPR was able to work with us to facilitate the project, but at the same time, not interrupt our business.”
Tera O’Hare, Senior Vice President of Technology for VGM Insurance, agreed. “SPR wowed everybody just like they have in the past,” O’Hare said of SPR’s initial approach – one that O’Hare said didn’t begin with machine learning (ML) but rather, about finding the best way to make better decisions.
“Machine learning is a little bit of a buzzword,” said O’Hare. “But it really wasn’t about the tool that we would use. It was about the ability to make this data work for us. For us, it’s about optimizing the process.”
With that as a goal, VGM and SPR got to work.
VGM’s Business Problem
VGM faced a big challenge: managing and predicting the risk associated with insurance claims.
The traditional methods relied heavily on manual processes and limited data analysis, which were not enough to handle the vast amounts of data VGM had. “VGM had a ton of historical data,” said Melissa McElroy, Senior Director of Solution Delivery at SPR. “We thought, if we look at your historical data, because we know already if there’s been a claim... we can look at the information about that policy. We already know if there’s a claim or not and that can help us figure out where these trends are. And then going forward we’ll gain more and more application data.”
The problem was that VGM’s systems and processes weren’t optimized to use their extensive historical data. This data was stored in different formats, like scanned documents, emails, and physical files, making it hard to extract and analyze. Because of this, VGM couldn’t fully leverage their data for predictive analysis, which is crucial for assessing claim risks.
“For us, it’s really about how we can optimize the processes and support what our underwriting team is already doing,” said O’Hare. “They have immense knowledge and expertise in this industry, but how do we give them these data elements that they’re having to go to ten different places to procure?”
Identifying the Solution
The project started with a hypothesis: “If we looked at historical application data, both new applications and renewals for insurance policies, we would be able to identify some characteristics about these clients that helped us understand their claim risk,” said McElroy.
SPR proposed a solution using optical character recognition (OCR) technology to digitize VGM’s historical data and apply machine learning models to predict claim risks. The process began with a comprehensive data extraction phase. Using OCR technology, SPR converted VGM’s vast repository of scanned documents and images into usable data formats. This step enabled the team to systematically analyze the historical data and identify patterns.
Pivoting the Approach
Initially, the team faced challenges as the data extracted from documents didn’t provide clear trends. “Most data science projects, machine learning projects, and AI projects, 70 to 80% of your time is spent trying to get the data set you need to build the model, said Bryan O’Connell, a software architect at SPR, after looking at the initial results, we had to make some changes to our approach to deliver that result they were looking for.” This led to a pivotal moment where the focus shifted from document data to client characteristics.
“What we realized as we went through our multiple iterations of this model is that it was the characteristics that were actually attached to the client themselves,” said McElroy. This pivot was a key learning point for the project. The team realized that while the document data was useful, the most significant predictors of claim risk were found in the client profiles.
This insight shifted the focus toward analyzing client attributes, like business type, revenue, and location, which were more indicative of potential claim risks. This adaptability and willingness to pivot were key to overcoming initial setbacks and moving toward a more effective solution.
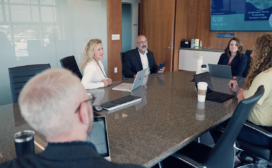
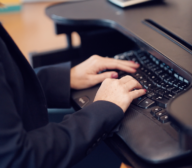
Structuring the Solution
SPR used Azure’s Document Intelligence Studio to digitize the historical data. This data was then used to train a machine learning model. The iterative process of developing and refining the model was cyclical: “You do the same six steps over and over again,” said O’Connell. “Prepare the data, build a model, measure the results, and come up with a new hypothesis.”
The ML model went through multiple iterations, each time refining its accuracy and predictive power. The team created seven different models, each focusing on various aspects of the data. Through these experiments, they identified the core attributes that significantly influenced claim risks. “This is a science experiment essentially,” McElroy said. “The approach is different than when we create other software.”
The final model categorized claim risks into different levels: low, medium, and high. This categorization was designed to be easily understood by business users, providing actionable insights. The integration of this model into VGM’s existing systems involved creating user-friendly interfaces that displayed these risk categories clearly. This approach ensured that the insights were not only accurate but also practical and useful for the VGM underwriting team to make decisions.
Technical Implementation
The implementation included developing an API that allowed VGM’s systems to communicate with the machine learning model seamlessly. “To put the model into production, we worked with VGM developers to integrate the model into their existing process,” O’Connell said. “We built an API for it that they could pass new data to and didn’t disrupt anything they were doing.”
This seamless integration ensured that the new system complemented VGM’s existing processes without causing disruptions. The categorization and scoring system provided a clear and actionable output for the underwriters, who could now make more informed decisions based on the model’s predictions.
“SPR brought some high performers,” said Hagberg. “They understood technology clearly where we don’t. They bring a skillset that we don't have on staff, or we don’t have capacity for. They did it times three.”
Impact on the Business
The implementation of the machine learning model had a profound impact on VGM’s business operations. “We’ve seen really great results thus far,” said O’Hare, noting that it not only streamlined the underwriting process but also provided valuable insights that helped VGM better understand their clients and manage risks more effectively.
The success of the project was evident in a multiple ways:
- Significantly improved the efficiency of the underwriting process. Underwriters could now assess claim risks more quickly and accurately, leading to faster decision-making.
- Provided deeper insights into client behaviors and risk factors, enabling VGM to offer more tailored and effective insurance solutions.
Collaborating and Communicating
The collaboration between the SPR and VGM teams was characterized by mutual respect, open communication, and a shared commitment to success. O’Hare reflected on the working relationship: “SPR’s ability to get up to speed very quickly from a business acumen standpoint, it’s of such value to the success of the project. It’s a true partnership versus just selecting a vendor.”
Both teams were committed to maintaining open lines of communication, regularly updating each other on progress, and being flexible in their approach. The two teams navigated the complexities of the project to achieve the desired outcomes. “SPR matched our intensity,” Hagberg said. “They came in right away, started delivering value, and were able to run. They were able to go step-by-step with our team at the level of performance we expect.”
Results
The positive impact of this project extends beyond immediate operational improvements. It has provided VGM with a robust framework for using data and advanced analytics in future projects. The ongoing collaboration with SPR continues to drive innovation and efficiency, positioning VGM as a forward-thinking leader in the insurance industry.
“Our first ML/AI project was incredibly successful in a number of ways,” said O’Hare. “The project was done on time, on budget. The future impact is this is how you go through one of these projects. So that’s the holistic value to VGM that our division got to drive.”
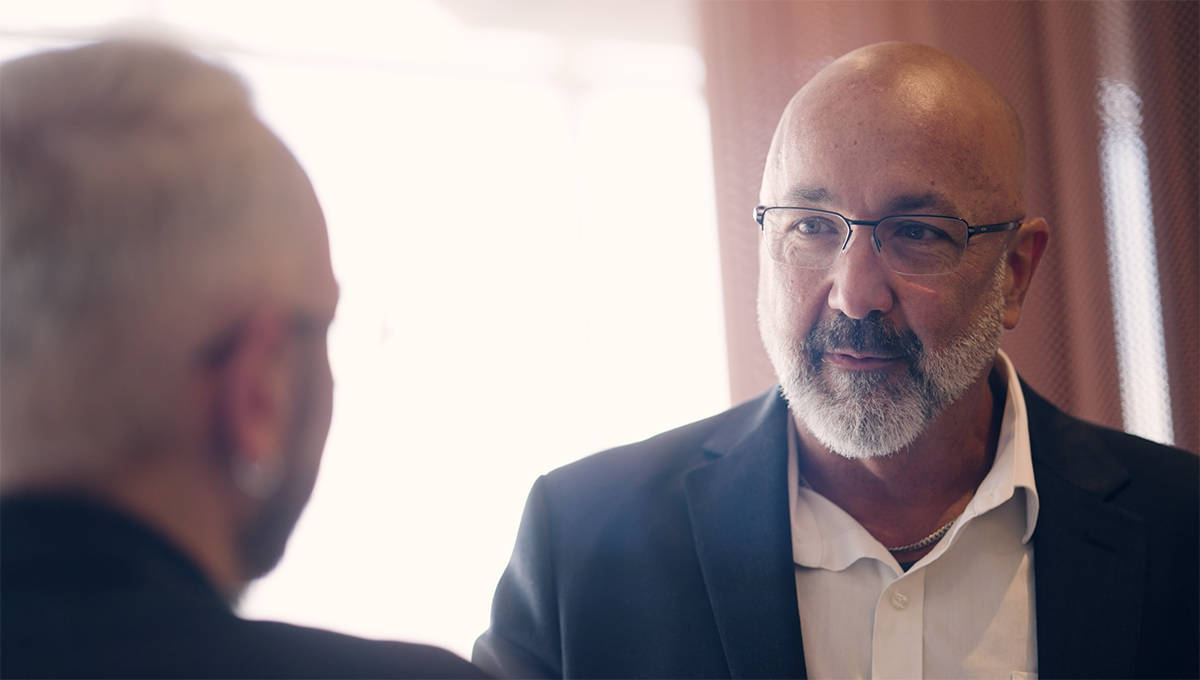
As Hagberg noted, whether you’re working with your own team, a consultant, a vendor partner, or a different business unit, there are challenges at the human level: “Working with SPR was as if we stood up the A-team,” Hagberg said. “Every interaction from SPR was seamless, making it no surprise that this project met its objectives in half the time on half the budget.”