AI/ML Projects: Understand the Complexities of Financial ROI
The advent of artificial intelligence (AI) and machine learning (ML) technologies has marked a significant milestone in the technological landscape, sparking interest and engagement from organizations globally. With advancements like ChatGPT and Large Language Models, these technologies are more relevant than ever before, making waves across various industry sectors.
However, as organizations embark on AI and ML journeys, they discover that calculating the financial return on investment (ROI) for these projects is not as straightforward as it is with traditional technology investments. The complexities cannot be calculated by simply subtracting expenses from revenue and dividing them by the total investment, but rather through estimating these figures with accuracy and foresight.
Let's take a look at the special considerations needed when projecting ROI specific to AI and machine learning projects. This blog will break down the components into revenue gains, expense reduction, and investments to demystify some of the intricacies involved. Whether you are an entrepreneur, a seasoned business leader, or someone intrigued by the financial aspects of these groundbreaking technologies, it's important to understand the unique challenges and opportunities ahead.
Revenue Gains: Enhancing Decision Making and Increasing Productivity
When it comes to revenue gains, AI and machine learning solutions can contribute to two key areas:
- Enhanced Decision-Making Capability: AI and ML can dramatically improve the accuracy and efficiency of decision-making processes.
- Increased Productivity & Expense Reduction: Implementing AI models can automate or expedite tasks without human intervention.
Expense Reduction: Human Capital Cost Savings
With the introduction of AI and ML solutions, expense reduction often comes in the form of reduced human capital costs. Employees no longer needed for tasks automated by AI can be repurposed for more complex roles or new revenue streams, further contributing to both expense savings and revenue gains.
An Example: The Loan Underwriting Process
Let’s use an example of the loan underwriting process for a bank. This bank processes thousands of loan applications a month, some of which are still on paper. In adopting AI and machine learning, the bank is likely to experience enhanced decision making during the underwriting process and during determination of interest rates of loans. The bank will also increase productivity by using AI and OCR to process their non-digital applications, currently done by hand. Since the bank no longer needs their employees to process handwritten applications or complete underwriting, these employees can focus somewhere else, further contributing to both expense savings and revenue gains.
Estimating Gains: Tips and Considerations
Productivity Increases and Expense Reduction: Estimating the productivity gains and expense reduction tends to be the more straightforward of these three components. For expense reduction, simply evaluate the human time and associated cost saved by implementing an AI solution. To estimate productivity gains, it is often sufficient to calculate the percentage increase enabled by the AI solution and the associated dollar amount with that increase. In our loan underwriting example, the productivity gains and expense reduction come from no longer having to process handwritten applications by hand.
Enhanced Decision Making: Due to the uncertainties around the effectiveness of models. Organizations should analyze current decision-making effectiveness and compare it with industry benchmarks. As with most things, it is much more difficult to go from good to great than it is from poor to good. In our example of loan underwriting, the enhanced decision-making would come from more appropriately assigning interest rates to loan risk, but how much more effective will the models be at estimating risk?
It is much easier to find what level of decision-making improvement would be required to achieve a reasonable ROI with all other variables held constant rather than to try and estimate the model’s enhanced decision capabilities before the model is built. Estimating model productivity is an extremely challenging task. It involves looking across the complexity and randomness of the problem at hand, the data inputs, and technical acumen. It is typically more productive to start with model building than it is to estimate how accurate your model will be. In the end, understanding how effective your models need to be to be useful for your organization is a valuable exercise even outside the context of ROI calculations.
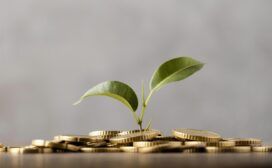
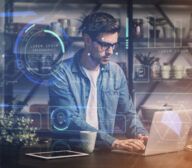
Total Investment
Estimating the total investment in an AI or machine learning solution is not too different from typical technical project estimation. There are some unique elements to consider as your organization is getting started, though.
The AI and Data Science Build Process: Unlike typical software engineering projects, which often follow a linear process, the AI and data science build process is highly iterative. Data scientists may experiment with various models, inputs, and technical approaches, constantly iterating to enhance subsequent models. This lack of predictability adds complexity to estimating both time and cost, making it a critical consideration.
Training the Models: A Unique Cost Aspect: Training AI models can be an expensive and computationally intensive process, depending on the model's size and complexity. The costs behind training models like ChatGPT, which experts estimate to be in the millions of dollars, serve as a stark example. Though not all models will require such substantial investment, the cost of training should be a vital consideration in your own projects.
Productionizing and Making Inferences: Integrating AI and machine learning tools into software systems or automated workflows is another significant investment. This includes not only the cost of integration but also the sometimes computationally intensive and costly process of making inferences with the models. Aligning the integration with organizational goals and budget constraints is essential.
Maintaining Systems: An Ongoing Commitment: AI and machine learning models require ongoing maintenance, as their performance may degrade over time due to changes in the world around them. The need to retrain and occasionally rearchitect models to maintain optimal performance adds another layer of complexity and cost to the investment.
Collaborating with Data Science Counterparts: Given these unique aspects, organizations must work closely with data science counterparts to understand the estimated costs across the entire lifecycle of AI solutions. Such collaboration ensures a more realistic and comprehensive understanding of the total investment.
AI and machine learning projects are undoubtedly at the forefront of technological innovation, offering organizations unparalleled opportunities for growth, efficiency, and competitive advantage. However, the complexities in projecting the return on investment for these initiatives are as unique as the technologies themselves. From the intricate considerations around revenue gains and expense savings to the multifaceted nature of total investment, AI and machine learning demand a nuanced understanding.
Embracing the iterative build process, acknowledging the substantial training costs, accounting for integration challenges, and preparing for ongoing maintenance are all essential to a comprehensive investment strategy.
In a time of rapid technological evolution, it is crucial for organizations to work closely with data scientists, industry experts, and strategic leaders to navigate the financial landscape of AI and machine learning projects. By demystifying the complexities, considering the full lifecycle of these solutions, and aligning with organizational goals, AI and machine learning can be more than just a technological marvel; they can become valuable assets driving growth and success.
The insights shared in this blog post are designed to guide decision-makers through the questions of AI investment. It is a path filled with challenges but also immense potential. With careful planning and special considerations, it is a journey that promises to shape the future of industries and redefine the way we do business. Whether just starting on this path or looking to optimize existing AI initiatives, understanding these special considerations is the key to unlocking the vast potential of AI and machine learning, transforming them from intriguing concepts into tangible business value.