ML Model Helps Staffing Company Retain Clients and Prevent Customer Churn
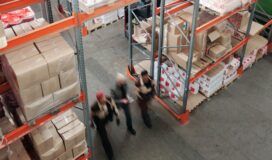
A staffing and recruiting company wanted a clearer view of their customers that might be at risk of leaving and partnering with a competitor. They considered machine learning as a solution and looked to SPR for advice on how to reach their goals.
Staying Current Through ML/AI
The staffing company provides pickers for warehouses and faces steep competition for the sites they staff. The team wanted to invest in a machine learning solution, as other teams within the company were already using machine learning and artificial intelligence. The client team also wanted a cloud-native solution. SPR advised on the appropriate tools and services within AWS that would help the client reach their goals.
SPR was brought in to create a proof of concept using their data set. When the client was impressed with the results of the proof of concept, SPR used AWS to formally deploy the model.
Many factors contribute to losing a client or a site churning or not churning, including:
- the local labor market
- regional unemployment
- logged communication activities in the client’s CRM
- how much they were billing their customer, and
- how much they were staffing for particular sites.
The model SPR created draws data from multiple sources, and data types include: socioeconomic data, HR data, CRM data, staffing information and Bureau of Labor statistics from government websites.
Automating the Process
Working with the client, SPR built a solution using AWS architecture. SPR set up the data pipelines and wrote the necessary code to transfer and transform the data residing in AWS. The new solution ingests data from multiple sources on a weekly basis and automatically reformats the data to work within the ML model. The model is automatically retrained each month using a Lambda function within SageMaker, so the model doesn’t degrade and results remain accurate. This automated process makes the model easier to maintain and reduces the amount of time spent in model tuning, data engineering and data reformatting.
The ML model allows the client to see the percentage chance that each site will churn and highlights different features and their relative importance at each site.
With this model running each week, the client can look at sites that are more likely to churn, examine what features are leading to that, and take action to remediate and keep the site.
Leadership now has a mechanism in place to really impact the evaluation and retention of their client sites. By analyzing the reports generated by the ML model, they can focus their efforts and change practices to be an even better business partner.